Research Statement
I want to further investigate deep learning from a statistical machine learning perspective. I want to better understand:
- The dynamics of training while using gradient descent methods on deep networks.
- The representative power of networks using particular architectures and specific datasets.
- Creating reasonable interpretations of decisions and predictions made by machine learning models for both field experts and novices.
In particular, I want to assess the interplay between training and validating and how the assumptions
we originally make align with the studied regimes of neural networks.
I believe these questions are fundamental to every nonconvex model we use and this path is key
to unlocking new potential of deep models' already incredible functionality.
Because I am interested in deep learning at such a fundamental level, I am interested in a
very broad array of application fields ranging across computer vision, climate science, computational biology, and deep reinforcement learning.
I am especially interested in methodologies which incorporate the structure of the data into the structure of the model
(e.g. convolutional networks, physics-informed machine learning, graph neural networks, causal structure learning, etc.)
Current Directions
My main research direction is on the study of "feature interactions", especially in the context of additive models. This is one of the primary candidates as a framework for bringing interpretability to deep learning. This direction formalizes any statistical interaction between existing features of a dataset and can be leveraged to explain the decisions of any blackbox model. In my research, I have been using this framework to explore both the interpretability and the robustness of general machine learning models.
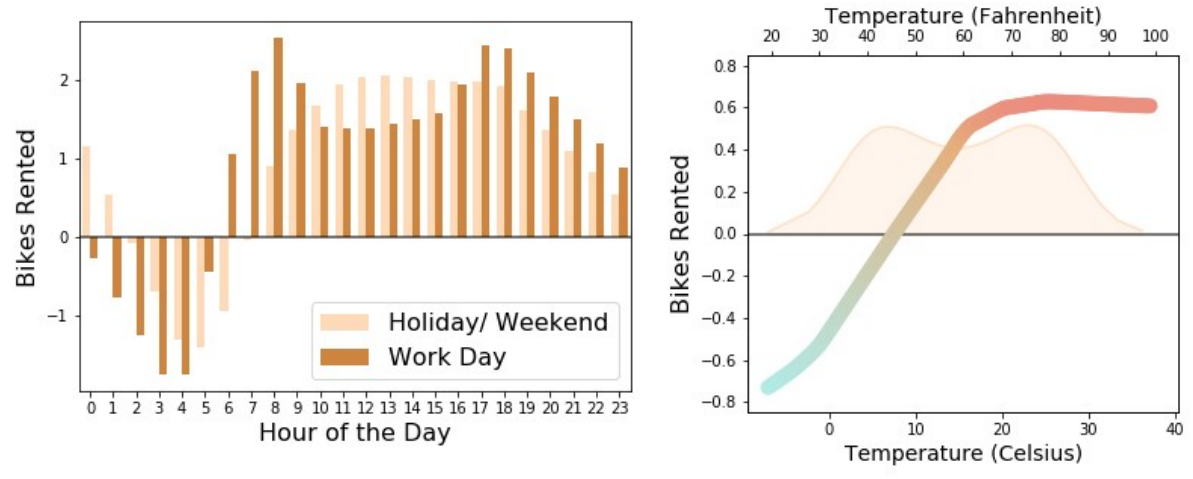
I have also applied these interpretable models in the causal inference setting for the study of fairness in an effort to understand why decision algorithms treat certain groups disparagingly and how this manifests in both treatment fairness and outcome fairness. Leveraging interpretability to understand these tradeoffs can help better manage algorithm fairness in practical settings.
My secondary research direction is about leveraging the vast amounts of existing human domain knowledge into the architectures we design for deep learning. Much like convolutional neural networks greatly accelerated the domain of computer vision, graph neural networks has enhanced the learning capabilities for molecule understanding, social network classification, and knowledge-graph understanding. I am primarily interested in leveraging physics-informed machine learning, simulation-informed machine learning, and causal-structure learning for understanding temporal structure and spatiotemporal data.
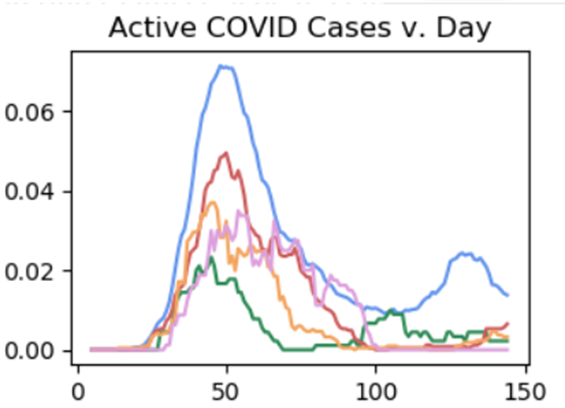
Previous Publications
[Neurips 2022]
Sparse Interaction Additive Networks via Feature Interaction Detection and Sparse Selection
[ICPR 2021]
Hierarchical Classification with Confidence using Generalized Logits
[ISVC 2019]
Hierarchical Semantic Labeling with Adaptive Confidence
Old Math Research
[Summer 2019] The Perfect Shuffle (Combinatorics)
[Summer 2018]
Signed Symmetric Chromatic Polynomial (Graph Theory)